Using machine learning algorithms combined with data analytics techniques to develop more efficient building
"by using machine learning algorithms combined with data analytics techniques , we could develop more efficient building designs based on energy consumption analysis"
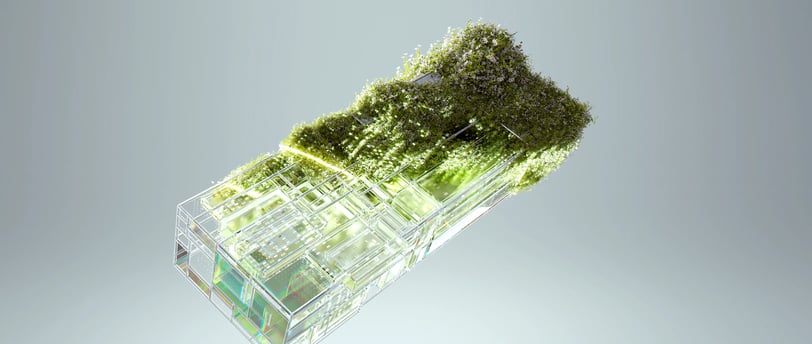
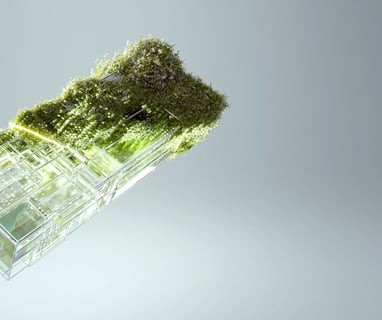
Introduction:
The efficient use of energy is an important consideration in building design. Buildings account for a significant portion of energy consumption, and as such, there is a need to develop more efficient building designs. In recent years, machine learning algorithms combined with data analytics techniques have emerged as a powerful tool for analyzing energy consumption patterns and developing more efficient building designs. This research aims to explore the potential of these technologies in improving building designs based on energy consumption analysis.
Section 1: Background
The efficient use of energy in buildings is becoming increasingly important due to concerns over climate change and the need to reduce our carbon footprint. In recent years, building design has focused on reducing energy consumption through the use of energy-efficient materials and systems. However, despite these efforts, energy consumption in buildings remains high.
One potential solution to this problem is to use machine learning algorithms combined with data analytics techniques to analyze energy consumption patterns in buildings and develop more efficient building designs.
Section 2: Machine Learning Algorithms and Data Analytics Techniques
Machine learning algorithms are a type of artificial intelligence that allows computers to learn from data and make predictions or decisions based on that data. Data analytics techniques, on the other hand, involve the use of statistical methods to analyze data and identify patterns and trends.
When combined, these technologies can be used to analyze large amounts of data on energy consumption in buildings, identify patterns and trends, and develop more efficient building designs.
Section 3: Examples of Machine Learning and Data Analytics in Building Design
There are several examples of machine learning and data analytics being used in building design. For example:
Predictive Maintenance: Machine learning algorithms can be used to predict when building systems are likely to fail, allowing for proactive maintenance to be carried out. This can prevent costly downtime and improve the energy efficiency of the building.
Energy Consumption Analysis: Data analytics techniques can be used to analyze energy consumption patterns in buildings and identify areas where energy use can be reduced. Machine learning algorithms can be used to develop predictive models that can be used to optimize energy use in buildings.
Fault Detection and Diagnostics: Machine learning algorithms can be used to identify faults in building systems, such as HVAC systems, and diagnose the cause of the fault. This can prevent energy waste and improve the energy efficiency of the building.
Section 4: Benefits of Machine Learning and Data Analytics in Building Design
The use of machine learning and data analytics in building design has several benefits, including:
Improved Energy Efficiency: By analyzing energy consumption patterns and identifying areas where energy use can be reduced, buildings can be designed to be more energy-efficient.
Cost Savings: Energy-efficient buildings can save money on energy bills, as well as reducing maintenance costs through predictive maintenance.
Improved Comfort: By optimizing building systems, such as HVAC systems, buildings can be designed to provide a more comfortable environment for occupants.
Reduced Carbon Footprint: By reducing energy consumption, buildings can contribute to the global effort to reduce carbon emissions and combat climate change.
Section 5: Challenges and Limitations
While the use of machine learning and data analytics in building design has several benefits, there are also challenges and limitations. These include:
Data Quality: Machine learning algorithms and data analytics techniques rely on high-quality data. Poor-quality data can lead to inaccurate predictions and decisions.
Cost: Implementing machine learning and data analytics in building design can be expensive, particularly for smaller buildings.
Complexity: Machine learning and data analytics are complex technologies that require specialized skills and knowledge to implement effectively.
Privacy and Security: The use of data analytics and machine learning in building design raises concerns about privacy and security, particularly with regards to the collection and storage of data.
Conclusion:
The use of machine learning algorithms combined with data analytics techniques has the potential to improve building design.